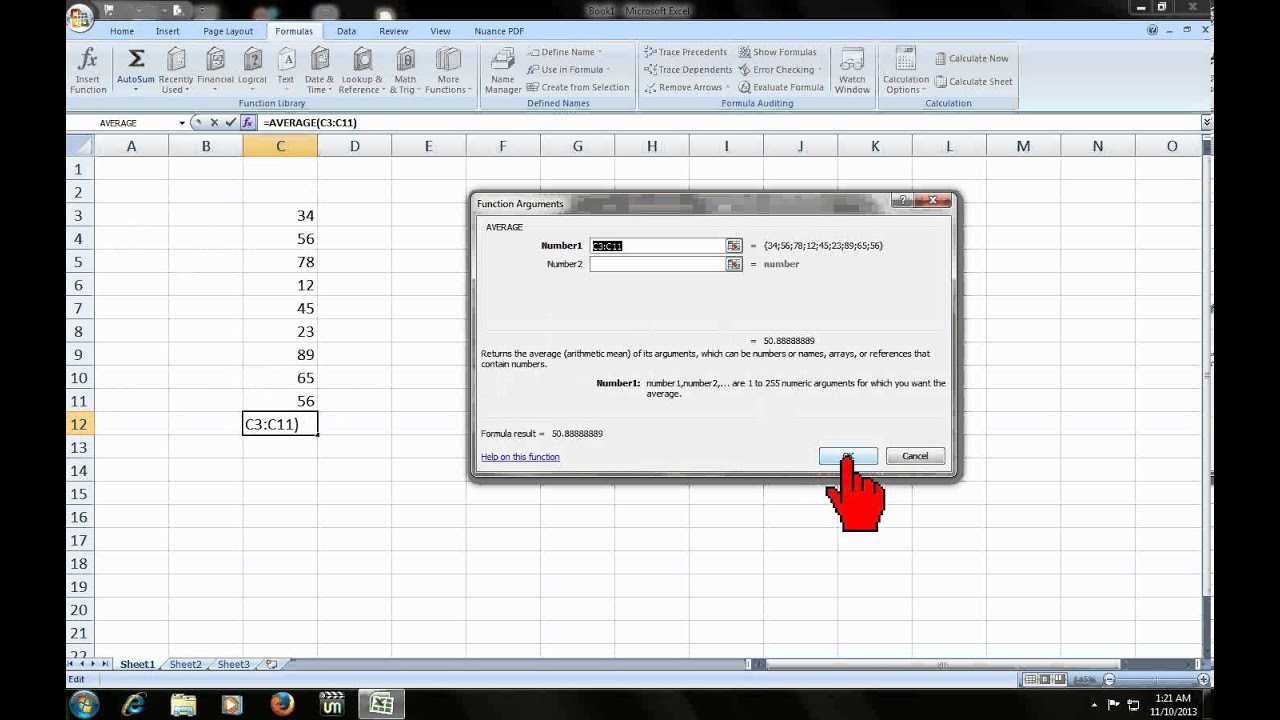
Are you struggling to find the p-value in Excel for your statistical analysis? Look no further!
In this article, we will guide you through the step-by-step process of finding the p-value using Excel functions. Understanding the importance of the p-value and interpreting the results for hypothesis testing will enhance your statistical analysis.
With our tips and tricks, you’ll become a pro at finding and analyzing p-values in Excel.
Understanding the Concept of P-Value
You should grasp the concept of p-value to fully understand statistical analysis.
The p-value is a measure that helps determine the significance of results in a statistical test. It’s a probability value that quantifies the likelihood of obtaining the observed data, or more extreme data, if the null hypothesis is true.
In simpler terms, the p-value tells you how likely it’s to obtain the observed results by chance alone. If the p-value is small (typically less than 0.05), it suggests that the observed results are unlikely to have occurred by chance, providing evidence against the null hypothesis.
On the other hand, if the p-value is large, it indicates that the observed results are more likely to be due to chance, therefore failing to reject the null hypothesis.
Understanding the p-value is crucial in statistical analysis as it helps in drawing valid conclusions and making informed decisions based on the results.
Importance of P-Value in Statistical Analysis
The p-value is a crucial measure in statistical analysis as it helps determine the significance of results and make informed decisions based on the data.
When conducting statistical analysis, it’s important to assess the likelihood that the observed results are due to chance. The p-value provides a quantifiable measure of this likelihood.
By comparing the p-value to a predetermined significance level, typically set at 0.05, you can determine whether the results are statistically significant or not. If the p-value is less than the significance level, it suggests that the observed results are unlikely to have occurred by chance alone, providing evidence in support of the alternative hypothesis.
On the other hand, if the p-value is greater than the significance level, it indicates that the observed results could have occurred by chance, leading to the acceptance of the null hypothesis.
In this way, the p-value helps researchers and decision-makers draw meaningful conclusions from their data and take appropriate actions.
Preparing Your Data for P-Value Calculation
Organizing your data is crucial for accurate p-value calculation. Before you can calculate the p-value, you need to ensure that your data is properly organized and formatted.
Start by arranging your data in a logical and clear manner. Make sure that each observation or data point is correctly labeled and entered into the appropriate columns or rows. Remove any unnecessary or duplicate data to avoid errors in your calculations.
Additionally, check for any missing values and decide on the best method for handling them, such as imputation or exclusion.
Once your data is organized, you can proceed with the p-value calculation, confident that your results will be reliable and accurate.
Step-by-Step Guide to Finding P-Value in Excel
Now let’s get into the step-by-step process of finding the P-value in Excel.
This guide will walk you through the calculations and interpretation of the significance level.
Excel P-Value Calculation
To find the P-value in Excel, you can follow a step-by-step guide.
First, you need to have your data ready in an Excel spreadsheet.
Next, you’ll use the T.TEST function to calculate the P-value. This function requires two arguments: the range of data for the first sample and the range of data for the second sample.
Once you enter the formula, press Enter to get the P-value. The P-value will be displayed in the cell where you entered the formula.
Remember that the P-value represents the probability of obtaining the observed data, assuming that the null hypothesis is true.
If the P-value is less than your chosen significance level (usually 0.05), you can reject the null hypothesis.
Excel makes it easy to calculate P-values, allowing you to analyze your data with confidence.
Significance Level Interpretation
You can interpret the significance level by comparing the P-value to your chosen threshold, usually 0.05, in order to make a decision about the null hypothesis.
The significance level, also known as alpha, is the probability of rejecting the null hypothesis when it’s actually true. If the P-value is less than or equal to the significance level, it means that the observed data is unlikely to occur if the null hypothesis is true. In this case, you’d reject the null hypothesis and conclude that there’s sufficient evidence to support the alternative hypothesis.
On the other hand, if the P-value is greater than the significance level, it means that the observed data is likely to occur even if the null hypothesis is true. In this case, you’d fail to reject the null hypothesis and conclude that there isn’t enough evidence to support the alternative hypothesis.
Interpreting P-Value Results for Hypothesis Testing
Now it’s time to understand the significance of the P-value results for hypothesis testing.
The P-value serves as a threshold to determine whether to reject or accept a hypothesis.
P-Value Significance Thresholds
Significantly, interpreting the results of the P-value for hypothesis testing is crucial in determining the significance thresholds.
The P-value represents the probability of obtaining results as extreme as the observed data, assuming that the null hypothesis is true. It’s a measure of the strength of evidence against the null hypothesis.
In hypothesis testing, a P-value of less than the significance level (usually set at 0.05) indicates strong evidence to reject the null hypothesis and support the alternative hypothesis. Conversely, a P-value greater than the significance level suggests weak evidence against the null hypothesis, and it isn’t statistically significant.
It’s important to note that the significance threshold should be determined before conducting the hypothesis test to avoid bias and ensure the validity of the conclusions drawn from the analysis.
Rejecting or Accepting Hypotheses
When interpreting P-value results for hypothesis testing, it’s important to consider whether to reject or accept the hypotheses.
The P-value represents the probability of obtaining the observed data, or more extreme data, assuming that the null hypothesis is true.
If the P-value is less than the predetermined significance level (usually 0.05), it suggests that the observed data is unlikely to occur if the null hypothesis is true. In this case, you’d reject the null hypothesis in favor of the alternative hypothesis.
On the other hand, if the P-value is greater than the significance level, it implies that the observed data is likely to occur even if the null hypothesis is true. In such instances, you’d fail to reject the null hypothesis.
It’s important to note that failing to reject the null hypothesis doesn’t necessarily mean that the null hypothesis is true; it simply means that there’s insufficient evidence to support the alternative hypothesis.
Common Mistakes to Avoid When Calculating P-Value
Are you making any common mistakes when calculating the P-value? It’s important to be aware of potential errors that can occur during this process.
One common mistake is misinterpreting the P-value. Remember, the P-value represents the probability of obtaining the observed data or more extreme data, assuming that the null hypothesis is true. It doesn’t indicate the probability of the null hypothesis being true or false.
Another mistake to avoid is using the wrong test statistic. Make sure you’re using the appropriate test statistic for your hypothesis test, whether it’s a t-test, chi-square test, or another type of test.
Additionally, double-check your sample size and data input to ensure accuracy. Mistakes in these areas can lead to incorrect P-values and ultimately flawed statistical conclusions.
Using Excel Functions for P-Value Calculation
To calculate the P-value in Excel, you can use specific functions and a coordinating conjunction to streamline the process. The two most commonly used functions are the T.DIST and T.DIST.2T functions.
The T.DIST function calculates the P-value for a one-tailed test, while the T.DIST.2T function calculates the P-value for a two-tailed test. Both functions require three arguments: the test statistic, the degrees of freedom, and the type of test.
The test statistic is the value calculated from your sample data, while the degrees of freedom depend on the sample size and the number of groups being compared. The type of test can be either 1 or 2, depending on whether it’s a one-tailed or two-tailed test.
Advanced Techniques for P-Value Analysis in Excel
Now let’s explore some advanced techniques for P-value analysis in Excel.
You’ll learn about Excel P-value formulas that can help you calculate P-values more efficiently.
It’s important to understand how to interpret P-values accurately to draw meaningful conclusions from your data.
Additionally, we’ll discuss common mistakes to avoid when working with P-values to ensure the validity of your statistical analysis.
Excel P-Value Formulas
You can utilize advanced Excel formulas to perform P-value analysis in Excel. These formulas allow you to calculate the P-value for different statistical tests, such as t-tests, chi-square tests, and ANOVA.
One commonly used formula is the T.DIST function, which calculates the probability of a value occurring in a t-distribution. This is useful when conducting t-tests to determine if there’s a significant difference between two groups.
Another formula is the CHISQ.TEST function, which calculates the P-value for a chi-square test. This is helpful in analyzing categorical data and testing for independence between variables.
Additionally, the ANOVA and F.DIST functions can be used to analyze variance and calculate the P-value for ANOVA tests.
Interpreting P-Values Accurately
Make sure to carefully analyze the compound noun in the P-value to accurately interpret its significance.
The P-value is a measure of the strength of evidence against the null hypothesis. It represents the probability of obtaining a test statistic as extreme as, or more extreme than, the observed value, assuming the null hypothesis is true.
In Excel, you can calculate the P-value using various statistical functions, such as T.TEST or Z.TEST. Once you have obtained the P-value, it’s crucial to interpret it correctly.
A P-value less than the chosen significance level (usually 0.05) suggests strong evidence against the null hypothesis, leading to its rejection. Conversely, a P-value greater than the significance level indicates weak evidence against the null hypothesis, leading to its acceptance.
Therefore, understanding the compound noun in the P-value is essential for making accurate interpretations and drawing valid conclusions from your statistical analysis.
Common P-Value Mistakes
Avoid the mistake of misinterpreting the P-value’s significance when conducting advanced techniques for P-value analysis in Excel.
One common mistake is treating a small P-value as evidence of a large effect size. Remember that the P-value only tells you the probability of obtaining the observed data or more extreme, assuming the null hypothesis is true. It doesn’t provide information about the size or practical significance of the effect.
Another mistake is interpreting a non-significant P-value as evidence of no effect. A non-significant P-value simply means that the data don’t provide strong evidence against the null hypothesis, but it doesn’t prove the null hypothesis to be true.
Always consider other factors such as effect size, sample size, and study design when interpreting P-values in Excel.
Comparing P-Values and Significance Levels
Understand the relationship between p-values and significance levels when comparing statistical data.
When conducting hypothesis tests, the p-value represents the probability of obtaining the observed data, or more extreme, if the null hypothesis is true.
On the other hand, the significance level, often denoted as alpha (α), is a predetermined threshold used to determine the level of evidence required to reject the null hypothesis.
In simple terms, the p-value measures the strength of the evidence against the null hypothesis, while the significance level sets the threshold for determining whether the evidence is strong enough to reject the null hypothesis.
It’s important to note that a p-value less than the significance level (usually 0.05) indicates statistical significance, suggesting that there’s enough evidence to reject the null hypothesis.
Enhancing Your Statistical Analysis With P-Value Insights
Improve your statistical analysis by gaining insights from the p-value.
The p-value is a crucial statistical measure that helps you determine the significance of your findings. By understanding the p-value, you can enhance your analysis and make more informed decisions.
One way to utilize the p-value is by comparing it to your significance level. If the p-value is lower than your significance level, it indicates that your results are statistically significant. This means that the probability of obtaining such results by chance alone is very low.
On the other hand, if the p-value is higher than your significance level, it suggests that your findings may not be statistically significant. By considering the p-value, you can determine the strength of your results and make more accurate interpretations in your statistical analysis.
Frequently Asked Questions
Can I Calculate the P-Value in Excel for Non-Parametric Tests?
Yes, you can calculate the p-value in Excel for non-parametric tests. Excel offers various statistical functions, such as T.TEST and NORM.S.DIST, which can be used to calculate p-values for different types of tests.
How Can I Handle Missing Data When Calculating the P-Value in Excel?
To handle missing data when calculating the p-value in Excel, you can use the appropriate function or tool to account for the missing values. This ensures accurate and reliable results for your statistical analysis.
What Should I Do if My Data Violates the Assumptions for Calculating the P-Value in Excel?
If your data violates the assumptions for calculating the p-value in Excel, you should consider using alternative statistical methods or adjusting your data to meet the assumptions before proceeding with the analysis.
Can I Calculate the P-Value in Excel for Multiple Comparison Tests?
Yes, you can calculate the p-value in Excel for multiple comparison tests. Excel offers various statistical functions that can help you calculate the p-value for different types of statistical tests.
Are There Any Limitations or Caveats to Keep in Mind When Interpreting P-Value Results in Excel?
When interpreting p-value results in Excel, keep in mind that there are limitations and caveats. These include the assumptions of the statistical test used, sample size, and potential sources of bias.
Conclusion
In conclusion, understanding how to find the p-value in Excel is crucial for conducting accurate statistical analysis.
By following the step-by-step guide and using Excel functions, you can easily calculate the p-value and interpret the results for hypothesis testing.
Additionally, advanced techniques can enhance your analysis and comparing p-values to significance levels provides valuable insights.
Incorporating p-value insights into your statistical analysis will help you make informed decisions based on the strength of evidence.